Difference between revisions of "DBP:HD"
m (Update from Wiki) |
m (1 revision imported: "pages" articles) |
(No difference)
|
Latest revision as of 23:53, 1 June 2017
Home < DBP:HDHUNTINGTON’S DISEASE
PI: Hans Johnson, Iowa University
Specific Aims
The NIH-funded project “Neurobiological Predictors of Huntington’s Disease” (PREDICT-HD) is studying Huntington’s disease (HD), a neurodegenerative genetic disorder that affects muscle coordination, behavior, and cognitive function, and causes severe debilitating symptoms by middle age. The aims of this DBP capitalize on two unique aspects of HD among neurodegenerative disorders, namely, the ability to know in advance exactly who will develop the disease and the knowledge that all affected individuals have the same root cause (i.e., a CAG repeat expansion in the huntingtin gene).
1. Perform individualized longitudinal shape change quantification from multimodal data. Morphometric brain differences begin 15 years or more before the symptoms of HD become debilitating. The ultimate goal of the PREDICT-HD study is to sufficiently define the neurobiological progression of HD in at-risk individuals so that clinical trials of potential disease-modifying therapies can be performed before symptoms reach a debilitating stage. Previous cross-sectional group analyses show that just before patients manifest symptoms, the caudate and putamen are severely affected [1] and there are widespread changes in cortical thickness [2, 3]. The NA-MIC multi-subject single modality group-wise registration methods will be modified to perform single subject multimodality longitudinal registrations.
2. Perform full brain diffusion tensor imaging tractography analysis. In addition to morphometric gray matter measurements, diffusion tensor imaging (DTI) fractional anisotropy measurements indicate that white matter changes occur very early in HD [3-5]. The development of tools for DTI data hold great promise for identifying early disease markers suitable for measuring longitudinal trajectory changes over short time intervals. Tools for segmenting white matter based on the high-resolution 3T multimodal scans plus DTI data consistently identify white matter anatomical regions. Methods for white matter fiber tracking from DTI data can identify anatomically connected regions within a single subject. Longitudinal analysis of white matter changes will help identify cause/effect relationships of disease progression between cortex, white matter, and sub-cortical connected regions.
3. Deploy extensible tools for sharing source data, derived data, algorithms, and methods to multi-site analysis teams. It is widely recognized that the PREDICT-HD imaging dataset has extraordinary value. It is further enriched through quality assurance documentation and integration with clinical measures. There is a great need to deploy mechanisms that facilitate external collaboration by providing: (1) data transfer methods for both raw scanner data and derived data, (2) access and interfaces to a wide variety of inter-connected image-processing algorithms from other institutions, (3) procedures for integration of externally generated derived datasets with the central repository, and (4) training material on how to best use the datasets.
Background
The PREDICT-HD (5 R01 NS04006) study is an international 30-site observational study of longitudinal neurodegeneration of persons at-risk for HD with continuous funding from 2001 to 2013. PREDICT-HD has fulfilled all aims from its initial award and has become part of a world-wide effort to provide treatments for HD, both symptomatic and presymptomatic (“premanifest”). The PREDICT-HD cohort and database have become international resources and offer an unprecedented opportunity to examine the pathophysiology and neurobiology of early HD. The specific short-term aims of PREDICT-HD are: (1) to refine the prediction of disease diagnosis (motor conversion) using longitudinal measures of plasma, imaging, cognitive performances, motor ratings, and psychiatric measures, and (2) to identify and characterize the natural history of sensitive markers of disease onset and progression that become abnormal prior to clinical diagnosis.
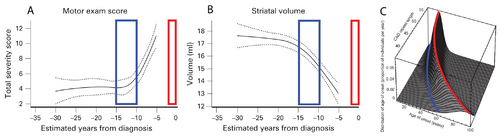
The curves in panels A and B of Figure 1 show a cross-sectional analysis of HD subjects, where the red boxes represent the most likely time of neurological diagnosis and the blue boxes represent the proposed window for starting a disease-modifying intervention [6]. A well-established parametric survival model [7], based on CAG repeat length, predicts the probability of observed debilitating motor neurological symptoms. This is the current basis for disease onset at different ages of individual patients. A graphical depiction of this “onset” model is shown in Figure 1C, where the red line indicates the most likely age at which a neurological diagnosis will be made.
The cross-sectional analyses that led to the results shown in Figure 1 are informative for identifying the general progression of disease, but individualized longitudinal analysis is needed to identify the appropriate time to start interventional treatment in the individual patient. The focus of this proposal is to improve the longitudinal analysis methods we currently use to include informed intervention criteria suitable for application decades before debilitating neurological symptoms manifest.
The PREDICT-HD study is currently collaborating with pharmaceutical companies to develop a promising long-term (3-10 year) therapeutic treatment for HD that involves a permanent implantable infusion pump for drug delivery. The NA-MIC Kit will contribute several technical aspects: (1) Precise subject-specific morphological mapping of white matter and gray matter sub-cortical regions will assist in developing the necessary masstransport models needed to optimize pump placement. (2) Longitudinal analysis of a single subject will inform clinicians of the most appropriate time for clinical intervention. (3) Finally, precision anatomical labeling will aid surgical planning for implantation.
Investigators
NAME | DEGREE | INSTITUTION | EXPERIENCE | ROLE |
HANS JOHNSON | PH.D. | UNIVERSITY OF IOWA | MEDICAL IMAGE PROCESSING, COMPUTER ENGINEERING & IMAGING INFORMATICS | DBP PI |
JANE S. PAULSEN | PH.D. | UNIVERSITY OF IOWA | NEUROPSYCHOLOGIST | PREDICT-HD PI |
VINCENT MAGNOTTA | PH.D. | UNIVERSITY OF IOWA | DIFFUSION TENSOR IMAGING, RADIOLOGY | CONSULTANT FOR DTI PROCESSING |
KENT WILLIAMS | M.S. | UNIVERSITY OF IOWA | SOFTWARE ENGINEERING, NA-MIC DEVELOPMENT BEST PRACTICES | DBP SOFTWARE ENGINEER |
DAN MARCUS | PH.D. | WASHINGTON UNIVERSITY, ST. LOUIS | NEUROIMAGING INFORMATICS | LEAD INFORMATICS & DATA DISSEMINATION |
MARTIN STYNER | PH.D. | UNIVERSITY OF NORTH CAROLINA | COMPUTER SCIENCE | LEAD ALGORITHM & ENGINEERING CONTACT |
Methods
Aim 1. To analyze longitudinal shape change, we will use a set of 80 subjects with between 3 and 6 longitudinal multimodal image sets (T1/T2/PD) and manually validated caudate, putamen, and thalamus segmentations to perform longitudinal registrations (i.e., not pairwise). This NA-MIC method will include provisions for addressing missing modalities. The proposed analysis will be used to provide robust estimates of longitudinal change. Additionally, the current NA-MIC shape analysis tools will be used to analyze a set of 225 subjects with between 3 and 6 T1 only scans and manually validated sub-cortical segmentations. These shape analyses will be used to create a normative model. In this way, changes in an individual’s scores can be used to inform clinical counseling and intervention scheduling decades before a neurological motor diagnosis is made.
The NA-MIC Kit 3D Slicer “Change Tracker” wizard provides functionality for investigating small longitudinal changes in pairwise bright object meningioma growth analysis. The “Change Tracker” is an exceptional prototype for the family of tools needed to analyze gray matter subcortical structure atrophy rates as a disease state marker. Development of the “Change Tracker” tools will be performed to generalize the tool for monitoring changes to subcortical brain structures.
Aim 2. The development of DTI quality control and atlas-building tools suitable for longitudinal white matter change quantification will be greatly accelerated by leveraging the existing NA-MIC expertise in DTI data processing. An HD-specific atlas will be constructed from a set 25 subjects with longitudinal 3T imaging data containing 2 high resolution T1, a high resolution T2, a 32 direction DTI sequence, and manually validated brain segmentations. We will collaborate with NA-MIC developers to create tools for a longitudinal analysis pipeline of changes measured by fiber tractography to identify white matter tracts that have strong co-morbid degenerative timelines compared to subcortical degeneration over time. We will use the same data for whole brain longitudinal analysis of the DTI connectivity using stochastic tractography tools for network and pathology detection. Customized user interfaces will extend the diffusion tractography visualization modules in the 3D Slicer to target reporting of longitudinal fiber tracking results to the clinical audience.
Aim 3. To accomplish the dissemination and collaboration goals, we will deploy the XNAT environment. This effort will include quality-assurance procedures, data-processing tools, and a common knowledge base accessible to the extended HD community of image-processing experts. The NA-MIC data-sharing tools will be extended to facilitate the dissemination of raw scan data, derived image datasets, and measurement scores for Aims 1 and 2. The existing morphometric analysis pipelines used to create the manually validated segmentations also will be incorporated into the XNAT processing pipeline.
Connections between this work and any of the other 3 proposed DBPs
The University of Iowa investigators have been long-term active participants in the development process for several NA-MIC Kit resources including ITK, XNAT, and 3D Slicer. The specific development needs of this DBP are consistent with many of the needs stated in the other DBPs. All of the projects have a need for robust well documented standardized workflows, longitudinal registration, and robust segmentation of anatomical regions. The HD, TBI, and RT for head and neck cancer DBPs share a common need for sensitive shape change measurement from longitudinal scans. Finally, the HD and TBI DBPs have a need for improved multi-model data analysis including quality control and analysis tools for investigating longitudinal white matter changes from diffusion tensor imaging.
Deliverables, Timeline, Impact
SPECIFIC AIMS | Year 1 | Year 2 | Year 3 |
Aim 1 | Preliminary tools for longitudinal shape change applied to existing sets of segmented subcortical structures | Improve shape analysis tools and apply to larger cohort with multiple study visits | Create normative models of shape change in healthy aging and disease (HD) |
Aim 2 | Quality control pipeline of DTI datasets. Test with preliminary tool for longitudinal analysis of white matter tracts | Put refined tool for longitudinal analysis of fiber tracts into workflow.
Application to subjects with apparent pathologic changes |
Develop workflow of optimized longitudinal white matter analysis for whole brain tractography |
Aim 3 | Stand up XNAT instance for PREDICT-HD project, customize to manage all expected data types.
Import existing data for internal use and testing |
Develop and incorporate PREDICT-HD specific workflows developed in Aims 1 and 2 into XNAT | Documentation of workflows with training materials. Enable sharing with the scientific community as dictated by the PREDICT-HD project. |
The Iowa investigators will collaborate with the Computer Science Core to develop new and refine existing tools to achieve the specific aims of the HD-DBP. This effort will include participation in the two All-Hands Meetings each year, as well as generation of presentations for scientific conferences and peer-reviewed publications. When mutually beneficial, tool development will be coordinated with other DBPs. Anonymized versions of the imaging datasets necessary to achieve the stated aims of this DBP will be made available to the entire NA-MIC community to facilitate algorithm, workflow, training, and documentation efforts. The developed tools will follow the software engineering Best Practices guidelines established by NA-MIC and will be made available through the Service and Dissemination Cores as well as the Neuroimaging Informatics Tools and Resources Clearinghouse. In the second and third years of the project, training events will be held to coincide with the Annual European Huntington’s Disease Network Meeting, and the Annual Human Brain Mapping Conference to expose the larger HD community to the NA-MIC resources. An XNAT instance will be deployed with the entire deidentified PREDICT-HD imaging dataset, representative standard workflows, and links to the clinical variables in the dbGaP will be made available to external researchers through a standard approval process. The NA-MIC efforts will facilitate our needs to: (1) integrate data from multiple protocols for generating a set of measures that can be studied to explore the longitudinal inter-relationships between known areas of degeneration, (2) disseminate a well documented set of best practices and training events for the HD imaging community to empower collaboration, (3) deploy a common centralized data-sharing infrastructure (i.e., XNAT) that is well integrated with the training software and development practices necessary to gain access to new imaging methodologies.
References
- Paulsen JS, Magnotta VA, Mikos AE, Paulson HL, Penziner E, Andreasen NC, et al. Brain structure in preclinical Huntington’s disease. Biol Psychiatry. 2006;59(1):57-6. PMID: 16112655.
- Nopoulos P, Magnotta VA, Mikos A, Paulson H, Andreasen NC, Paulsen JS. Morphology of the cerebral cortex in preclinical Huntington’s disease. Am J Psychiatry. 2007;164(9):1428-34. PMID: 17728429.
- Rosas HD, Hevelone ND, Zaleta AK, Greve DN, Salat DH, Fischl B. Regional cortical thinning in preclinical Huntington disease and its relationship to cognition. Neurology. 2005;65(5):745-7. PMID: 16157910.
- Reading SA, Yassa MA, Bakker A, Dziorny AC, Gourley LM, Yallapragada V, et al. Regional white matter change in pre-symptomatic Huntington’s disease: a diffusion tensor imaging study. Psychiatry Res. 2005;140(1):55-62. PMID: 16199141.
- Beglinger LJ, Nopoulos PC, Jorge RE, Langbehn DR, Mikos AE, Moser DJ, et al. White matter volume and cognitive dysfunction in early Huntington’s disease. Cogn Behav Neurol. 2005;18(2):102-7. PMID:15970729.
- Paulsen JS, Langbehn DR, Stout JC, Aylward E, Ross C, A, Nance M, et al. Detection of Huntington’s disease decades before diagnosis: the Predict-HD study. J Neurol Neurosurg Psychiatry. 2008;79:874-80. PMID: 18096682.
- Langbehn DR, Brinkman RR, Falush D, Paulsen JS, Hayden MR. A new model for prediction of the age of onset and penetrance for Huntington’s disease based on CAG length. Clin Genet. 2004;65(4):267-77. PMID: 15025718.