Difference between revisions of "Projects:DTI DWI QualityControl"
Line 6: | Line 6: | ||
[[Image:DTIPRep GUI.png|400px|thumb|right| DWI-based QC results using DTIPrep through three steps: 1) converting dicom to nrrd format of DWI image, 2) loading the protocol and running the software and 3) potential needed of visual checking and final saving. In this example, gradient #11 suffers from intensity artifact and is excluded. The sphere shows 3D view of gradients distribution before and after running DTIPrep in blue and green colors respectively. ]] | [[Image:DTIPRep GUI.png|400px|thumb|right| DWI-based QC results using DTIPrep through three steps: 1) converting dicom to nrrd format of DWI image, 2) loading the protocol and running the software and 3) potential needed of visual checking and final saving. In this example, gradient #11 suffers from intensity artifact and is excluded. The sphere shows 3D view of gradients distribution before and after running DTIPrep in blue and green colors respectively. ]] | ||
− | [[Image: | + | [[Image:GradientsDistribution.png|400px|thumb|right|3D view of gradients distribution with different b-values. ]] |
As theoretical work characterizing DTI grows, it is essential to increase its practical usability from a clinical environment perspective. Inherently, DWI images suffer from a vast variety of artifacts and the acquisition time for diffusion MRI is longer than conventional MRI due to the need for multiple acquisitions to obtain directionally encoded Diffusion Weighted Images (DWI). This leads to increased motion artifacts and reduced signal-to-noise ratio (SNR). Therefore, in a clinical environment, this imaging technique needs additional processes such as appropriate QC assessment methods to increase its practical usability. We are developing a framework, called DTIPrep, for assessing and correcting DWIs and DTI. | As theoretical work characterizing DTI grows, it is essential to increase its practical usability from a clinical environment perspective. Inherently, DWI images suffer from a vast variety of artifacts and the acquisition time for diffusion MRI is longer than conventional MRI due to the need for multiple acquisitions to obtain directionally encoded Diffusion Weighted Images (DWI). This leads to increased motion artifacts and reduced signal-to-noise ratio (SNR). Therefore, in a clinical environment, this imaging technique needs additional processes such as appropriate QC assessment methods to increase its practical usability. We are developing a framework, called DTIPrep, for assessing and correcting DWIs and DTI. |
Revision as of 16:45, 2 January 2013
Home < Projects:DTI DWI QualityControlBack to UNC Algorithms
Diffusion Tensor and Diffusion Weighted Imaging Quality Control
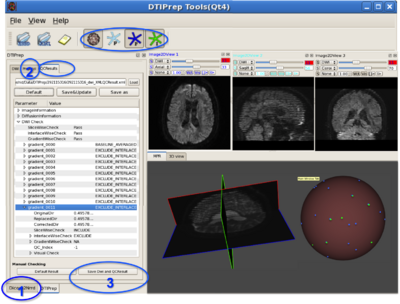
As theoretical work characterizing DTI grows, it is essential to increase its practical usability from a clinical environment perspective. Inherently, DWI images suffer from a vast variety of artifacts and the acquisition time for diffusion MRI is longer than conventional MRI due to the need for multiple acquisitions to obtain directionally encoded Diffusion Weighted Images (DWI). This leads to increased motion artifacts and reduced signal-to-noise ratio (SNR). Therefore, in a clinical environment, this imaging technique needs additional processes such as appropriate QC assessment methods to increase its practical usability. We are developing a framework, called DTIPrep, for assessing and correcting DWIs and DTI.
Description
Current framework for DWI QC
DTIPrep is the first comprehensive and fully automatic pre-processing tool for DWI and DTI quality control can provide a crucial piece for robust DTI analysis studies. The protocoling, reporting, visual controlling and data correction capabilities are used to produce high consistence and inter-rater reliable QC results. This framework is organized by pipeline steps include: 1) image info checking, 2) diffusion info checking, 3) Slice-wise intensity checking, 4) Interlace-wise intensity checking, 5) Averaging baseline images, 6) Eddy current and motion correction, 7) gradient-wise checking of residual motion/deformations, 8) Computing DTI measurements and saving and 9) Optional visual QC.
Ongoing extension using DTI QC
In our ongoing research, we are extending our QC procedures to include a QC step based on the derived DTI data. This step detects and potentially removes residual artifacts that are not commonly detected in the individual DWIs. In our large scale population studies, we observed several such artifacts, most specifically an artifact of "dominating direction" (see figure to the right). In order to detect such artifacts, we propose a new approach via the entropy of the Principal Direction (PD) histogram computed over the major region of the image (e.g. the full brain). Given a prior knowledge of expected entropy values for acceptable scans, the quality of the DTI image is categorized into acceptable, suspicious and highly suspicious/rejection categories using calculated the standard scores.
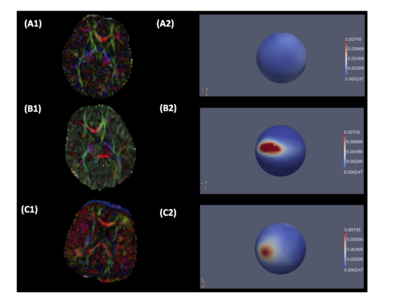
This approach can also be employed to correct data: Using a leave-one-out scheme, we iteratively determine the best candidate DWI image for exclusion as the one with optimal improvement in the corresponding DTI's entropy measure. This removal of DWI's continues until either DTI image is not longer classified in the rejection class.
Publications
Key Investigators
- UNC Algorithms: Mahshid Farzinfar, Zhexing Liu, Martin Styner, Clement Vachet
- Utah Algorithms: Tom Fletcher, Ross Whitaker, Guido Gerig, Sylvain Gouttard
Links
- DTIPrep on NITRC